Weekly Research Paper Summary on Machine Learning - #4
Written on
Chapter 1: Overview of Selected Papers
This week, I will delve into three significant research papers within the field of machine learning.
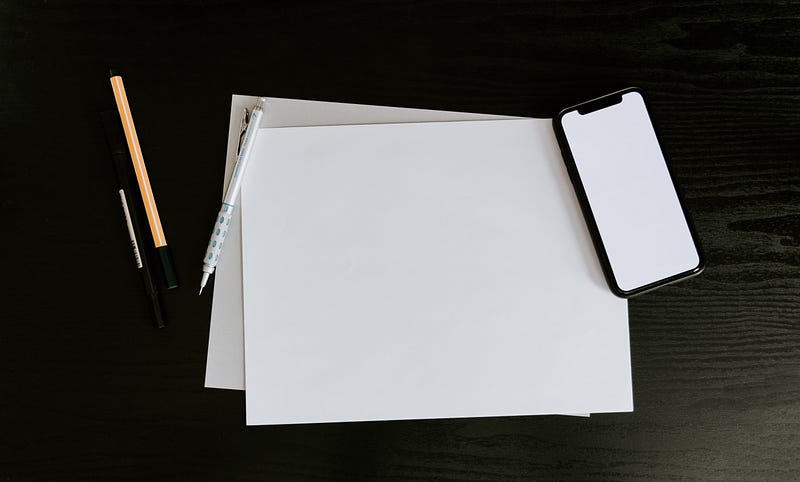
Section 1.1: Scalable Outlying-Inlying Aspects Discovery
Authors: Nguyen Xuan Vinh, Jeffrey Chan, James Bailey, Christopher Leckie, Kotagiri Ramamohanarao, and Jian Pei
Venue: Pacific-Asia Conference on Knowledge Discovery and Data Mining 2015 (PKDDD 2015)
Paper: URL
Abstract:
This research addresses the challenge of outlying aspects mining, focusing on how certain features can highlight the uniqueness of a query object. Recent studies have approached this through two main strategies: (i) feature selection techniques that identify the most distinguishing features between a query point and the rest of the dataset, and (ii) score-and-search methods that define an outlyingness score and explore subspaces where the query point performs best. This paper introduces OARank, a hybrid framework that combines the efficiency of feature selection with the effectiveness of score-and-search methods, proving to be significantly faster than previous approaches and well-suited for large datasets.
Section 1.2: High Contrast Subspaces for Density-Based Outlier Ranking
Authors: Fabian Keller, Emmanuel Muller, and Klemens Bohm
Venue: 2012 IEEE 28th International Conference on Data Engineering (ICDE 2012)
Paper: URL
Abstract:
Outlier detection is a crucial aspect of data analysis, where outliers are defined as objects that significantly differ from their local surroundings. This paper highlights the limitations of existing density-based ranking methods, which often yield random results due to insufficient contrast between outliers and normal objects. It proposes a novel method for identifying high contrast subspaces that enhance outlier ranking, presenting a new metric for evaluating subspace contrast. Results demonstrate that this approach surpasses traditional dimensionality reduction techniques and improves outlier ranking quality.
Section 1.3: A Neural Data Structure for Novelty Detection
Authors: Sanjoy Dasgupta, Timothy C. Sheehan, Charles F. Stevens, and Saket Navlakha
Venue: Proceedings of the National Academy of Sciences of the United States of America (PNAS)
Paper: URL
Abstract:
The challenge of novelty detection is crucial for organisms to differentiate new stimuli from previously encountered ones. This study reveals that the olfactory circuit of fruit flies has evolved a variant of a Bloom filter to evaluate odor novelty. Unlike a standard Bloom filter, this biological system adjusts its responses based on the similarity to past odors and the time since their last encounter. The research outlines a predictive framework for the novelty responses of fruit flies and extends these insights to create advanced Bloom filters that outperform traditional models in various biological and computational scenarios.
Chapter 2: Previous Reading Lists
For those interested in exploring earlier discussions, check out my previous weekly reading lists: #1, #2, #3.
About the Author
I am Durgesh Samariya, currently in my third year of a Ph.D. program in Machine Learning at FedUni, Australia. Online, I am recognized as TheMLPhDStudent.
Stay updated by subscribing to my newsletter for weekly insights.
Connect with me online:
- Kaggle
- GitHub
- Medium
Thank you for reading!