Revolutionizing Healthcare: Machine Learning Applications Explained
Written on
Chapter 1: Introduction to Machine Learning in Healthcare
In this discussion, we explore the transformative potential of machine learning within the healthcare sector. Understanding how machine learning functions in this context is crucial for grasping its significance. This article aims to provide a comprehensive overview of current applications of machine learning in healthcare, highlighting real-world examples that enhance patient outcomes and minimize expenses.
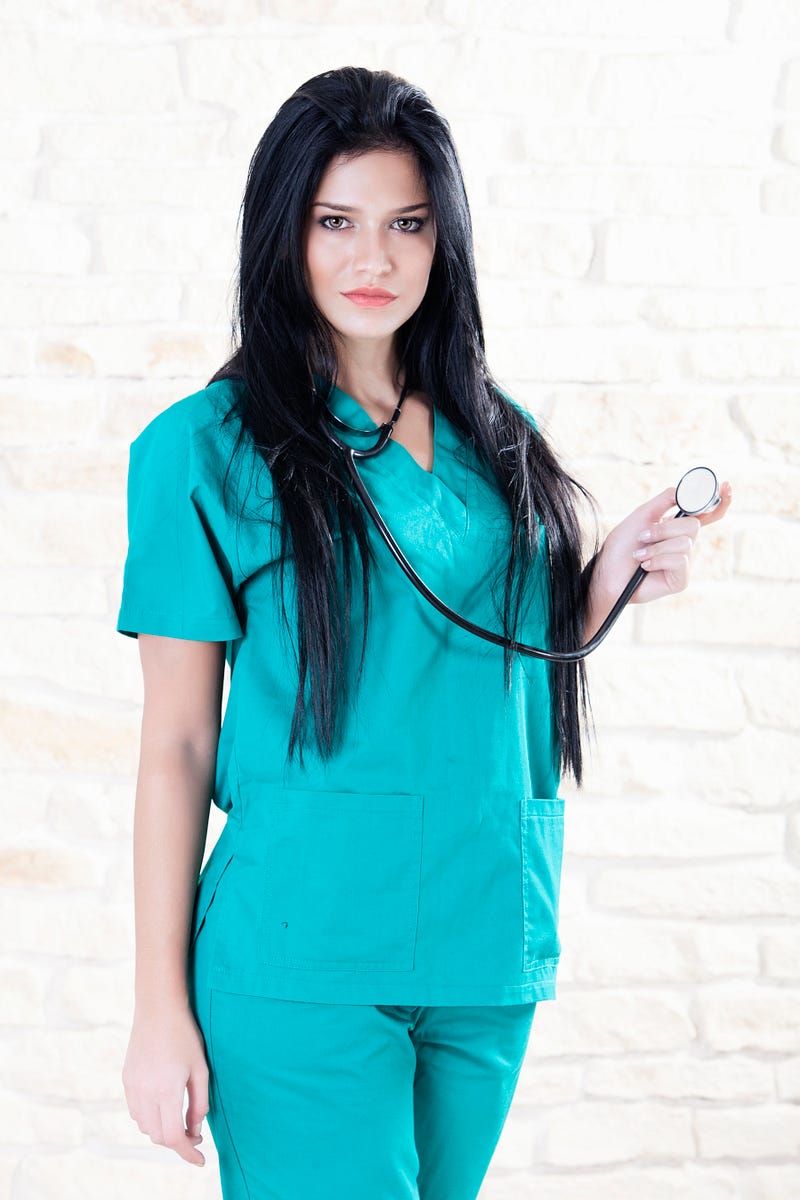
Chapter 2: The Role of Machine Learning in Medical Diagnostics
Machine learning is increasingly integrated into the medical domain. As a subfield of artificial intelligence, machine learning employs algorithms that learn from data to forecast future outcomes. As technology progresses, its applications span various industries, particularly in healthcare, where it assists in diagnosing conditions and tailoring treatments to individual patient needs.
Section 2.1: Diagnosing Health Conditions
Hospitals like Stanford University Medical Center and The Mayo Clinic have utilized machine learning for diagnosing conditions such as diabetes and various cancers. These institutions implement deep neural networks that simulate human brain functions, enabling more accurate cancer detection than traditional methods.
This video titled "Machine Learning in Health Care" discusses how these technologies are reshaping patient care and improving diagnostic accuracy.
Section 2.2: Enhancing Drug Discovery and Production
The drug discovery process, often lengthy and costly, can significantly benefit from machine learning. This technology optimizes manufacturing processes, manages drug supplies, and employs predictive analytics to avert shortages. For instance, Deep Genomics uses AI to accelerate drug design, reducing the timeframe from four years to just four weeks.
Section 2.3: Advancements in Medical Imaging
Medical imaging plays a crucial role in diagnosis and disease monitoring. Machine learning and deep learning facilitate automated image analysis, providing clinicians with enhanced diagnostic precision. By training models on extensive datasets, machine learning algorithms can identify patterns indicative of tumor growth and differentiate between benign and malignant tumors.
This video, "Machine Learning for Population Health," further explores how machine learning techniques are applied in medical imaging to improve diagnostic outcomes.
Chapter 3: Patient Data Analytics and Personalized Medicine
Section 3.1: Utilizing Patient Data for Better Outcomes
Machine learning tools analyze healthcare data to identify trends and anomalies, aiding in proactive decision-making. Predictive analytics can forecast patient outcomes based on historical data, allowing for timely interventions that prevent unnecessary hospitalizations.
Section 3.2: Tailoring Treatments with Personalized Medicine
Personalized medicine focuses on customizing healthcare based on individual patient data, contrasting with traditional evidence-based approaches. Machine learning plays a vital role in predicting treatment responses, especially in oncology, where specific drugs may be more effective for certain tumor types.
Chapter 4: Conclusion
Machine learning's influence on healthcare is profound, offering numerous advantages that enhance medical practice. With capabilities ranging from accurate diagnoses to efficient drug discovery and personalized treatment plans, machine learning is poised to revolutionize patient care. As we harness these technologies, the future of healthcare looks brighter, promising improved efficiency, reduced costs, and better patient outcomes.