Harnessing Simulation Techniques for Data Mastery
Written on
Understanding Simulation in Data Science
In the realm of data science, it's often beneficial to conduct a rehearsal using a fabricated yet plausible dataset prior to gathering, purchasing, or analyzing actual data. This process is referred to as simulation.
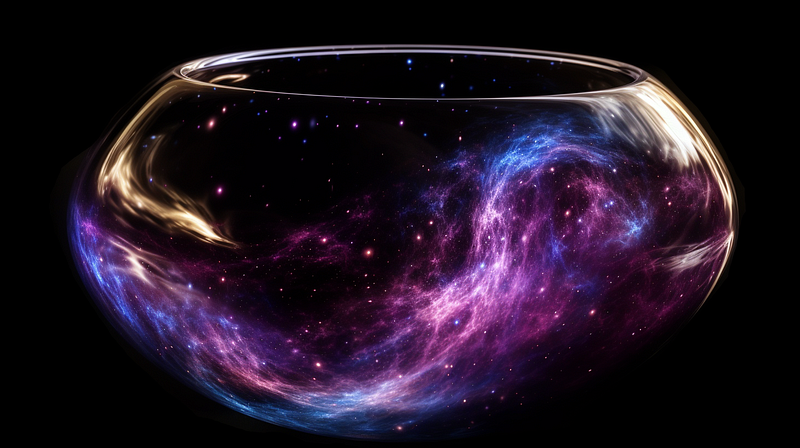
Image by the author.
(Note: The links in this article lead to further explanations by the same author.)
Typically, simulation is performed using random number generators in popular data processing tools like Python or R. By employing random distribution functions, you can create observations based on any characteristics you desire. If this sounds unfamiliar, think of it as programming a computer to flip a coin, roll a die, or generate lottery numbers—though the complexity can be tailored to your specifications.
The videos below provide a demonstration of this process.
Understanding how simulation works is akin to how generative AI models produce compelling text and visuals. While the foundational distributions are much more intricate than a simple rnorm(1000) in R, the principle remains similar: writing a prompt for generative AI is essentially sampling from a complex distribution. Although it may seem advanced, the rapid advancements in computer hardware and capabilities have outpaced traditional educational methodologies.
What about data analysts who prefer using spreadsheets and shy away from coding? (While I encourage you to explore coding, here’s a non-code perspective.) Simulation offers a fantastic opportunity to craft your own scenarios and define your own parameters.
For instance, if you're a spreadsheet enthusiast planning to gather coffee tasting data, rather than consuming a lot of coffee only to realize that the data collected is unmanageable, you can simulate your data by creating a column in a spreadsheet with values like “good, good, gross, gross, gross.” You can adjust the length of this column as desired, testing different sizes to validate your hypotheses. Through this exercise, you might discover that certain lengths yield insufficient data to support your conclusions, a situation known as an underpowered study. Finding this out before actual experimentation could save you from a regrettable situation.
Additionally, if you think you might be collecting irrelevant data, it’s advantageous to identify the necessary data points—like the time of day each cup was tasted—before engaging in extensive coffee consumption. Recording this information after sampling multiple instances might leave you feeling unwell, which could lead to an undesirable repeat of the process.
As you consider incorporating simulation into your data gathering and analytical strategies, take a moment to explore how to optimize your rehearsals.
Thanks for reading! If you're interested in expanding your knowledge, check out my YouTube course designed for both novices and seasoned professionals.
P.S. Have you ever clicked the clap button on Medium multiple times to see the outcome? If you enjoy the content, feel free to connect with me on Twitter, YouTube, Substack, and LinkedIn. If you're interested in having me speak at your event, please use this form to reach out.